Discovering new subtypes of breast cancer using artificial intelligence
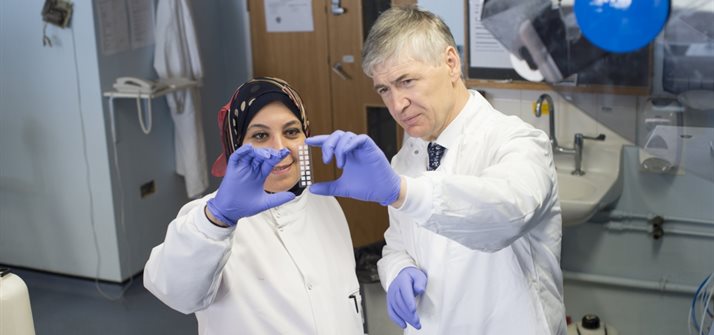
Breast cancer is the most common cancer and cause of cancer death in women in England. In 2003, 36,500 new cases of breast cancer were diagnosed, representing 32% of all cancers in women. Out of these, 10,500 women died, a rate of 29 deaths per 100,000 women.
Breast cancer is a heterogeneous group of diseases, with complex and distinctive underlying molecular pathogenesis.To address the breast cancer disease heterogeneity, clustering approaches have become more and more popular, especially for discovering profiles in cancer with respect to high-throughput genomic data. In literature, protein biomarker panels have been applied, with known relevance to breast cancer, to large numbers of cases using tissue microarrays, exploring the existence and clinical significance of distinct breast cancer classes through clustering approaches.
However, since different clustering algorithms result in different clusters, particularly when large multi-dimensional data sets are considered, consensus clustering methodologies have been used in recent studies.
At the Advanced Data Analysis Centre (ADAC), analysts have applied different clustering algorithms to a large panel of patients presented at Nottingham City Hospitals with primary operable breast cancer, and, through a consensus clustering approach, have identified possible novel cancer subtypes. These subtypes have been subsequently refined by the ADAC centre by developing artificial intelligence techniques for biomedical decision support.
In particular, fuzzy logic has been used to model the imprecision and uncertainty inherent in medical knowledge representation and decision making. The resulting seven groups of the breast cancer disease now need external clinical validation, but this research contributed to the creation of the so-called NPI+, a new clinical tool which modifies the original NPI by including modern 'genetic' markers. By doing so, the (proven) power of the original NPI is refined and enhanced to provide personalised advice to patients.